An Action-Packed Computer Vision System
SMAST is an advancement in AI that enables computer vision systems to track objects in space and time, and understand their relationships.
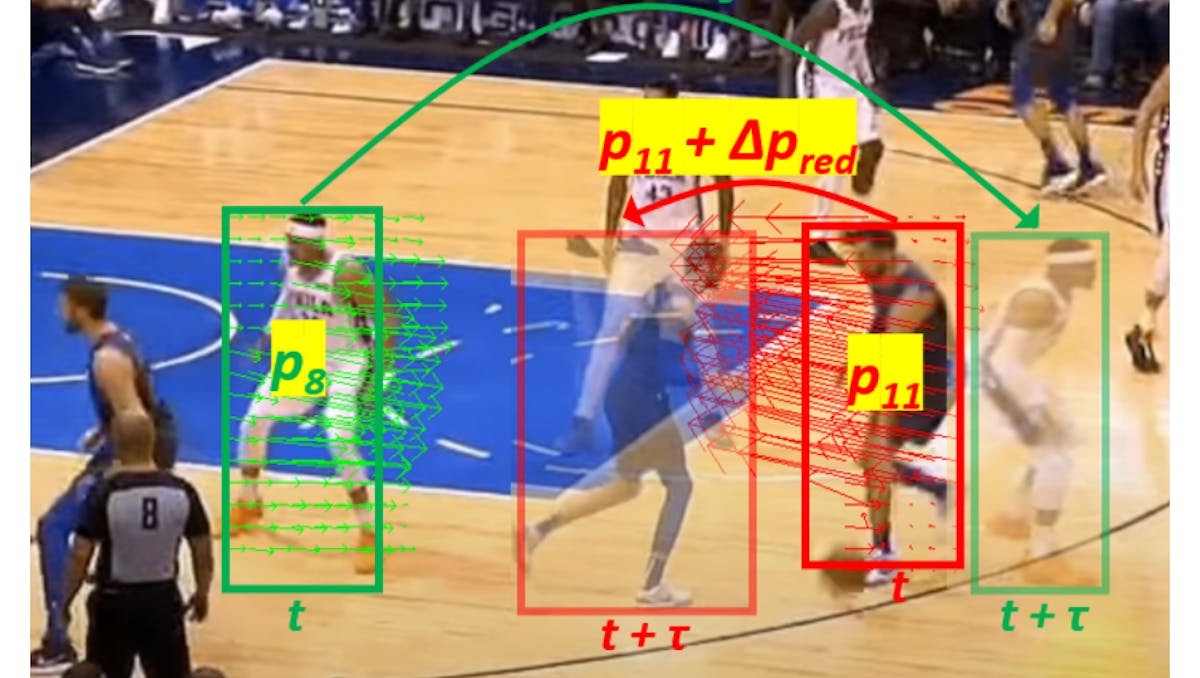
On the long and winding path that leads artificial systems to an understanding of their surroundings, one of the first stops is the recognition of specific objects in a video stream. This is a crucial hurdle to clear, and many existing algorithms are capable of doing quite a good job of it. But to get the more complete understanding that the next generation of applications will need, these computer vision systems will have to dig much deeper. They must understand how these objects move through time and space, and how they interact with one another.
However, most present technologies struggle with the complexity of spatiotemporal interactions between action semantics, such as the relationship between persons and objects in dynamic scenes. While earlier approaches like motion trajectory tracking captured some aspects of object movement, they often fail to account for the crucial interactions between all action elements, such as the interplay between a person and a ball in a kicking action.
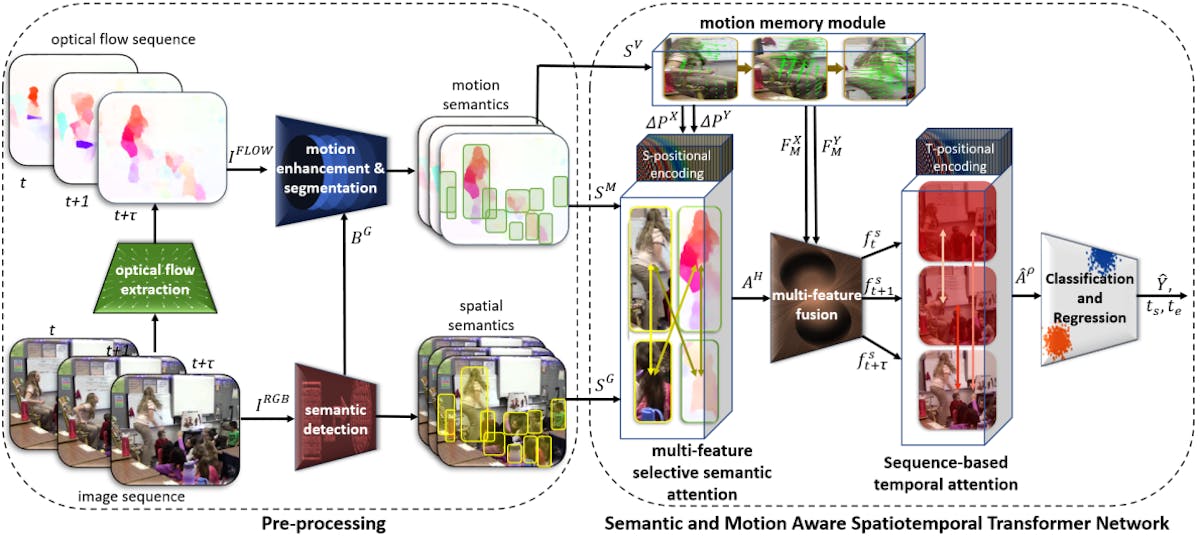
Additionally, temporal dependencies between action frames pose a significant challenge. Actions unfold sequentially but often in a temporally heterogeneous manner — some actions require focusing on adjacent frames, while others depend on understanding relationships between keyframes that are far apart in time (e.g., the start, middle, and end of a jump). Traditional methods like RNNs are biased toward adjacent frames and lack the flexibility to capture these diverse temporal dependencies. Even transformer networks, while more advanced, are still biased toward similar and adjacent frames, limiting their ability to fully capture the non-adjacent temporal relationships that are critical in many actions.
Engineers at the University of Virginia have put forward a new solution to this problem that they call the Semantic and Motion-Aware Spatiotemporal Transformer Network (SMAST). It contains a novel spatiotemporal transformer network that improves the modeling of action semantics and their dynamic interactions in both the spatial and temporal dimensions. Unlike traditional approaches, this model incorporates a multi-feature selective semantic attention mechanism, which allows it to better capture interactions between key elements (e.g., persons and objects) by considering both spatial and motion features. This addresses the limitations of standard attention mechanisms, which typically focus on a single feature space and miss the complexities of multi-dimensional action semantics.
SMAST also features a motion-aware two-dimensional positional encoding system, which is a significant improvement over standard one-dimensional positional encodings. This new encoding scheme is designed to handle the dynamic changes in the position of action components in videos, making it more effective in representing spatiotemporal variations. The model also includes a sequence-based temporal attention mechanism, which can capture the diverse and often non-adjacent temporal dependencies between action frames, unlike previous methods that overly emphasize adjacent frames.
By addressing these gaps, SMAST not only improves the efficiency of processing action semantics but also enhances the accuracy of action detection across various public spatiotemporal action datasets (e.g., AVA 2.2, AVA 2.1, UCF101-24, and EPIC-Kitchens). Experiments revealed that this approach consistently outperforms other state-of-the-art solutions.
R&D, creativity, and building the next big thing you never knew you wanted are my specialties.