Diffusing GenAI Confusion
Weird generative AI images got you down? ElasticDiffusion takes a different approach to eliminate extra fingers, legs, and other oddities.
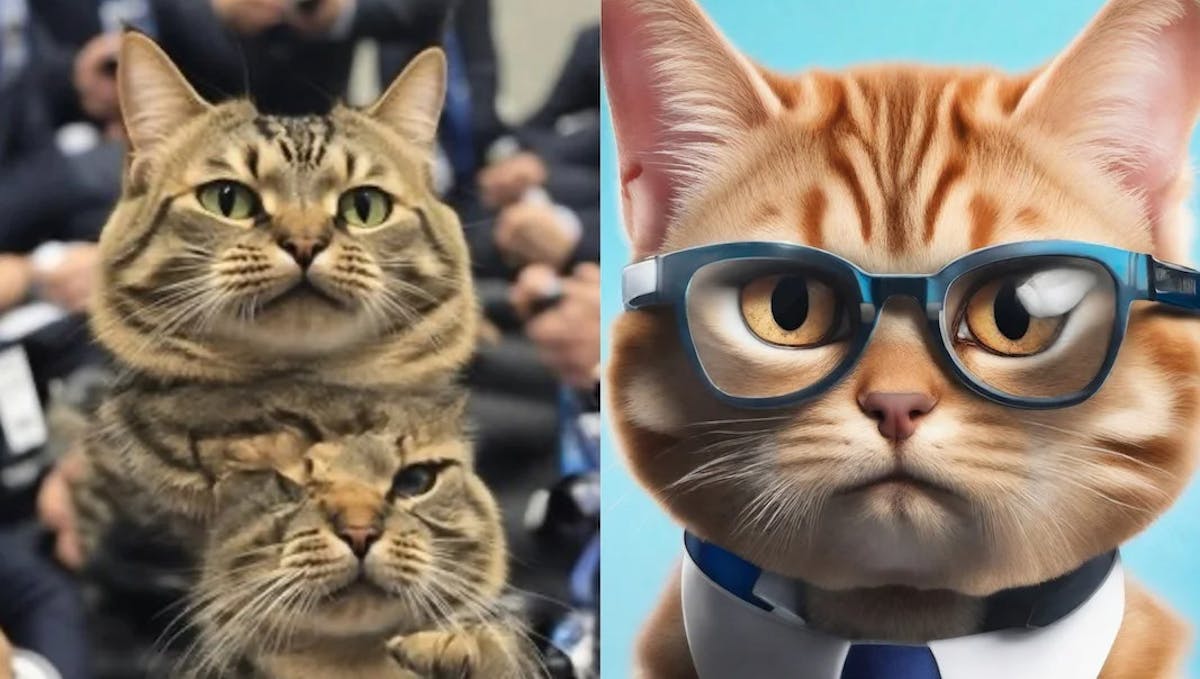
One of the most prominent applications to arise from the recent artificial intelligence boom is the text-to-image generator. These tools allow a user to request what they would like to see via a text prompt, which they then turn into an often strikingly good graphical representation of that request. This capability has been made possible by advances like the development of the diffusion model, which most famously powers the Stable Diffusion algorithm.
Anyone that has ever used these tools knows that while they are very powerful and useful, they can also do some very odd things. For example, when asking for images of people it is not uncommon that they are generated with extra fingers or legs, or in poses that defy the laws of physics or just plain old common sense. For these reasons, the products of today’s text-to-image generators are often immediately identifiable and of limited use for many applications.
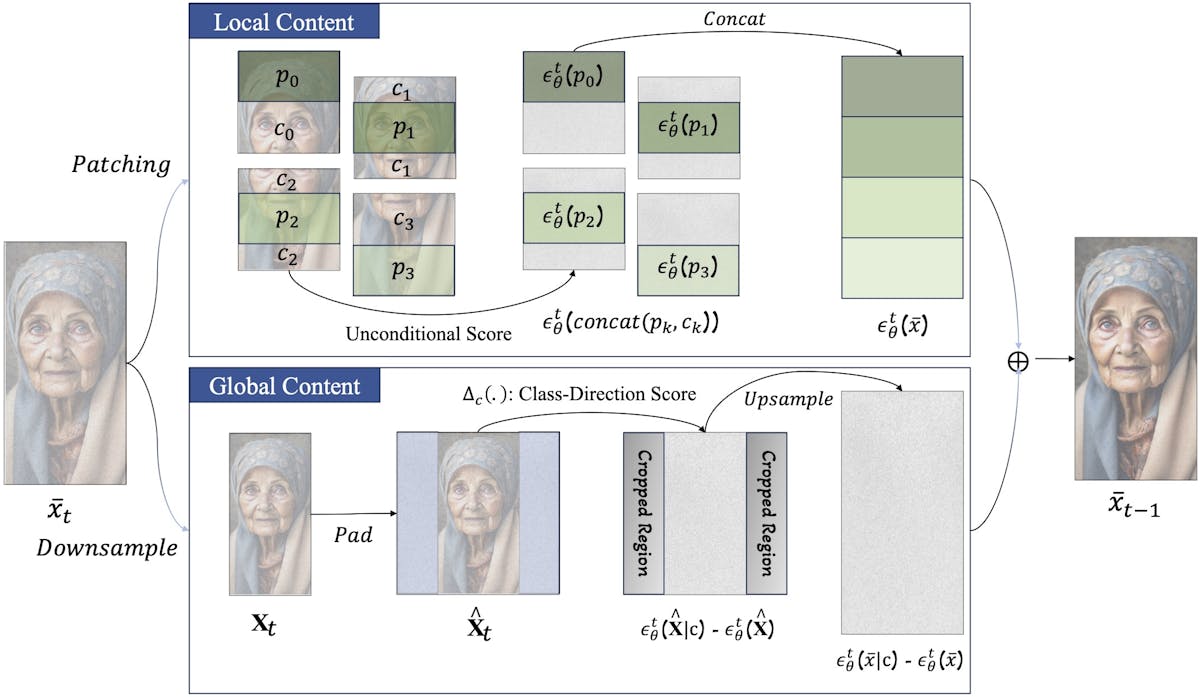
These oddities arise from the way that diffusion models operate and the way that they are trained. During training the algorithms are shown examples of many images, all of a given resolution, to learn how to associate elements of images with textual descriptions. Then when a user requests a new image from a trained model, it begins with many layers of random noise. That noise is gradually removed in an iterative process as the image is refined. But if the request is well outside of the distribution of the training data, or if the size of the generated image must differ from it, things can start to go wrong quickly.
In theory, these problems might be solved by training the models on larger datasets. But given the massive volumes of data that cutting-edge algorithms are already trained on — and the associated costs — that is not a very practical solution. But a team of researchers at Rice University came up with another option called ElasticDiffusion. This approach takes steps to avoid weirdness in the generated images without requiring unreasonable amounts of training data.
When a diffusion model is converting the image’s initial noisy state into a finished product, it takes both a local and global approach. Local updates operate at the pixel level to fill in fine details, like textures and small objects. Global updates, on the other hand, sketch out much more broad structures, like the overall shape of a person. Because these operations typically happen together, these models are not very adaptable and struggle to generate coherent images under suboptimal conditions — like when the resolution of an image needs to differ from the training data.
But with ElasticDiffusion, local and global updates are independent. First the global updates are made, filling in the overall layout and structure of the image. Then pixel-level local updates are made to the image, one tile at a time. This approach has been demonstrated to produce cleaner, more coherent images without repeating elements or other oddities. And ElasticDiffusion does not require any additional training data to achieve these results.
At present, the team’s work may not be terribly attractive to users as the algorithm takes up to nine times longer to run than other options like Stable Diffusion and DALL-E. But they are actively working to improve performance, so in the near future we may be able to generate more convincing synthetic images.
R&D, creativity, and building the next big thing you never knew you wanted are my specialties.