Looking to the Future of Smart Glasses
ElectraSight enables ultra-low-power, real-time eye tracking for smart glasses by using non-invasive QVar sensors and on-device tinyML.
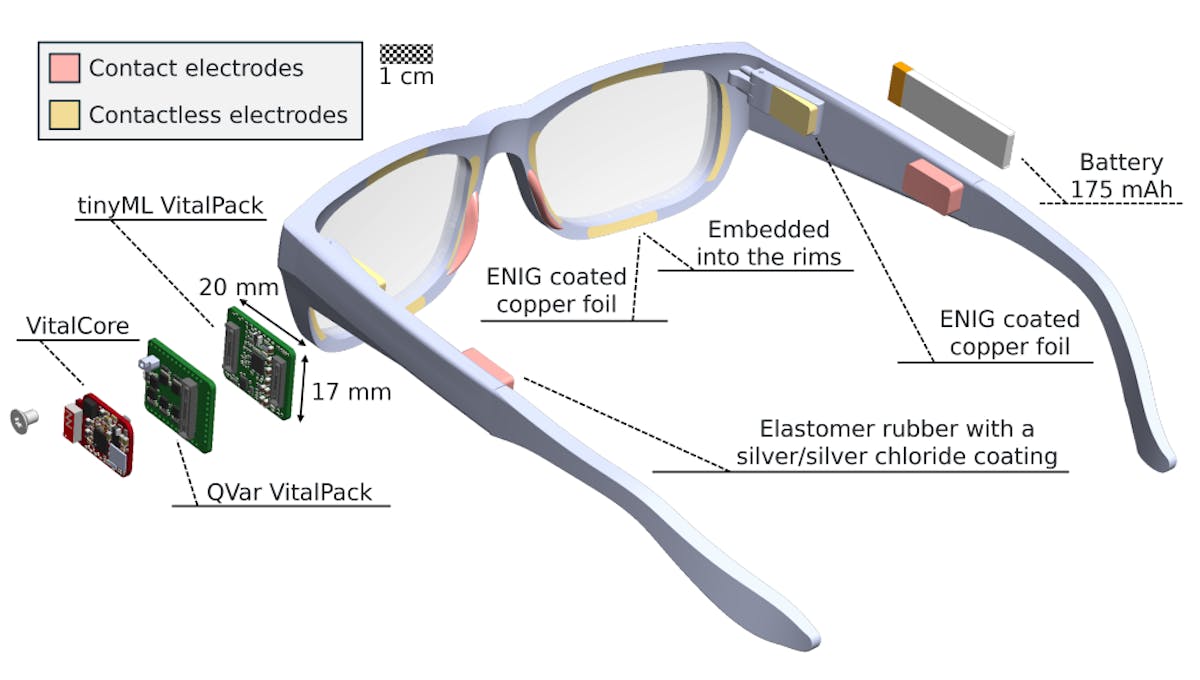
Smart glasses are getting smarter all the time, and that is giving us new ways to interact with our digital devices and the world around us. When it comes to smart glasses, one of the most efficient and natural ways to interact with them is through eye movements. By tracking where we are looking, these devices can give us contextually-relevant information about our surroundings or allow us to navigate digital interfaces without ever needing to touch a screen or use voice commands. For instance, a simple glance at an object could pull up information about it, such as the price of an item in a store or historical facts about a monument.
But for this to be possible, smart glasses must be equipped with an eye-tracking mechanism. This is frequently handled by camera-based systems. These tend to be highly accurate, yet they are often bulky and consume a lot of energy, making them impractical for deployment in the frames of glasses. Furthermore, always-on cameras raise a lot of privacy-related issues that can hinder adoption of the technology.
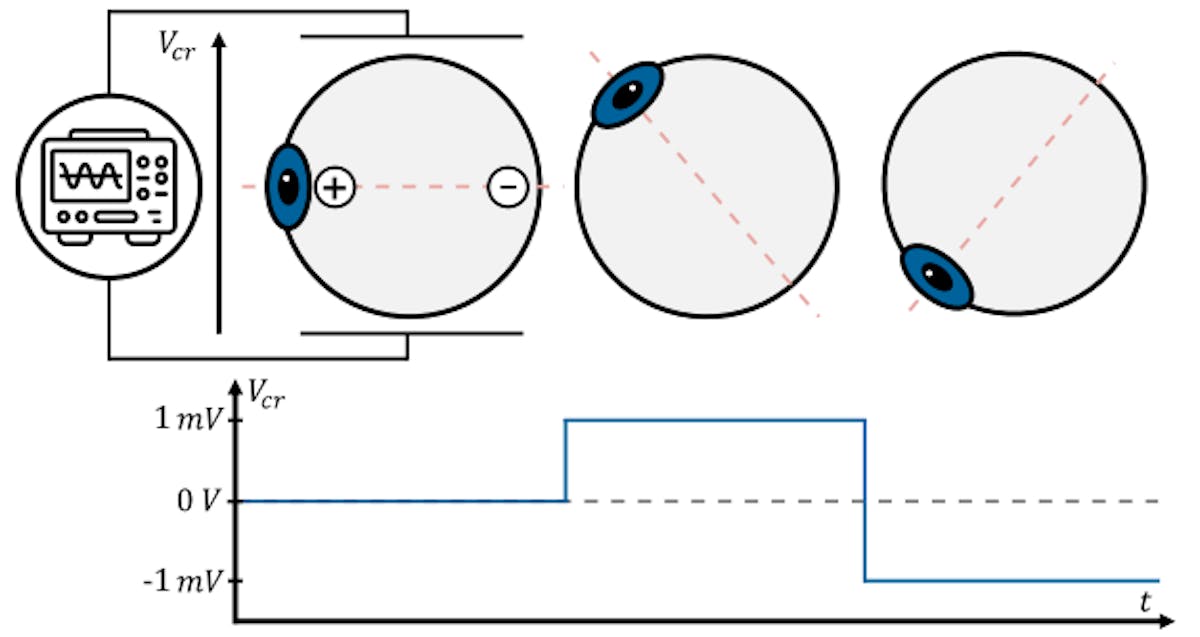
Electrooculography (EOG) solves the problems associated with camera-based technologies, but it provides far less detailed and accurate information. Recently, a team at ETH Zurich has developed a hybrid contact and contactless EOG system that is non-invasive and can run directly onboard smart glasses. Unlike previous EOG-based solutions, the team’s approach, called ElectraSight, is highly accurate.
The hardware platform for ElectraSight is an ultra-low-power system designed to enable non-invasive eye tracking through capacitive and electrostatic charge variation sensing. The platform incorporates advanced QVar sensors, such as the STMicroelectronics LSM6DSV16X and ST1VAFE3BX, which use high-impedance differential analog front ends to detect the corneo-retinal potential — bioelectric signals generated during eye movements. These sensors are characterized by their low noise levels, high sensitivity, and efficient power consumption, with the ST1VAFE3BX offering programmable gain, a high sampling frequency of up to 3,200 Hz, and a total current consumption of just 48 µA.
The platform is built around three modular components: the VitalCore, tinyML VitalPack, and QVar VitalPack. The VitalCore serves as the central node, powered by the Nordic Semiconductor nRF5340 system-on-chip, which integrates a dual-core Arm Cortex-M33 processor, Bluetooth 5.2, and extensive GPIO interfaces within a compact footprint. The tinyML VitalPack incorporates a GAP9 microcontroller, a high-performance, low-power processor designed for edge AI tasks, featuring RISC-V cores and a neural engine optimized for deep learning operations. This coprocessor handles the computationally intensive tasks of real-time eye movement classification.
The QVar VitalPack hosts six ST1VAFE3BX sensors for flexible multi-channel sensing, enabling various electrode configurations and contactless sensing. The system is designed for integration, with SPI-based communication between the nRF53 on the VitalCore and the QVar sensors, ensuring efficient data acquisition through direct memory access. Data is processed in predefined windows and forwarded to the GAP9 coprocessor for real-time analysis.
An accompanying tinyML model leverages 4-bit quantized convolutional neural networks to classify eye movements in real time with good accuracy — 92 percent for six classes and 81 percent for ten classes — without requiring calibration or user-specific adjustments. The model operates within just 79 kB of memory, making it highly efficient for deployment on resource-constrained hardware platforms. Experimental results demonstrated that ElectraSight is able to deliver low latency performance, with 90 percent of movements being detected within 60 ms and real-time inferences completed in just 301 µs.
The team has also produced a comprehensive dataset of labeled eye movements. This data can be used to evaluate the performance of future eye-tracking systems, and they hope it will move the ball forward in the research and development of smart glasses.
R&D, creativity, and building the next big thing you never knew you wanted are my specialties.