Making a Splash in Autonomous Drone Adaptability
Liquid neural networks allow this autonomous drone to adapt to drastically different environments with no special training.
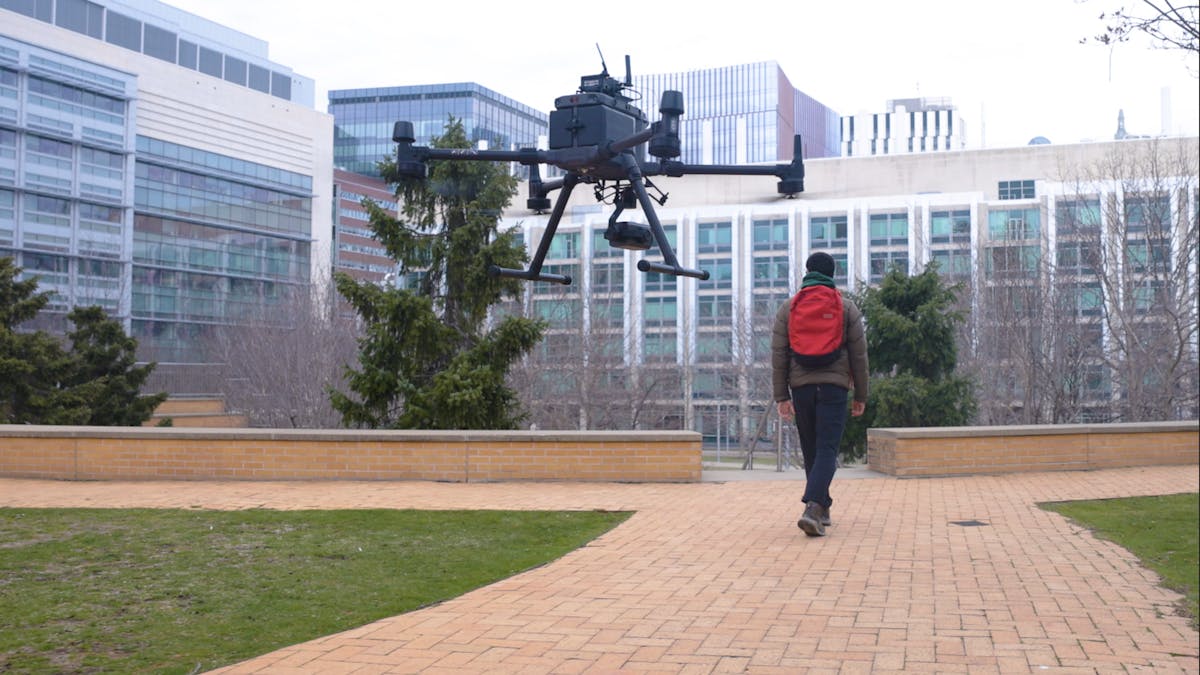
Artificial intelligence has come a long way in recent years, with neural networks and other machine learning algorithms becoming more advanced and capable than ever before. However, one of the biggest challenges facing AI researchers today is the development of a generalized neural network that can adapt to new environments. This problem is particularly significant in the development of self-driving cars and autonomous drones, where the ability to quickly and accurately adapt to changing conditions is crucial.
The challenge lies in the fact that neural networks are typically designed to perform a specific task that is learned from a limited set of training data. For example, a neural network designed to recognize objects in a photograph might work well in a lab setting where the lighting is controlled, but could struggle when faced with the varying lighting conditions and different backgrounds that might be encountered in the real world. Similarly, a self-driving car might be able to navigate a predefined route with ease, but could encounter difficulties when faced with unexpected obstacles, differing weather conditions, or changes to the route.
Researchers at MIT’s CSAIL have recently published the results of their effort to improve the adaptability of neural networks to new environments. They applied their methods to an autonomous drone that was built for vision-based fly-to-target tasks. The key to the system’s adaptability to previously unseen environments lies in the use of a relatively new development in the field — liquid neural networks.
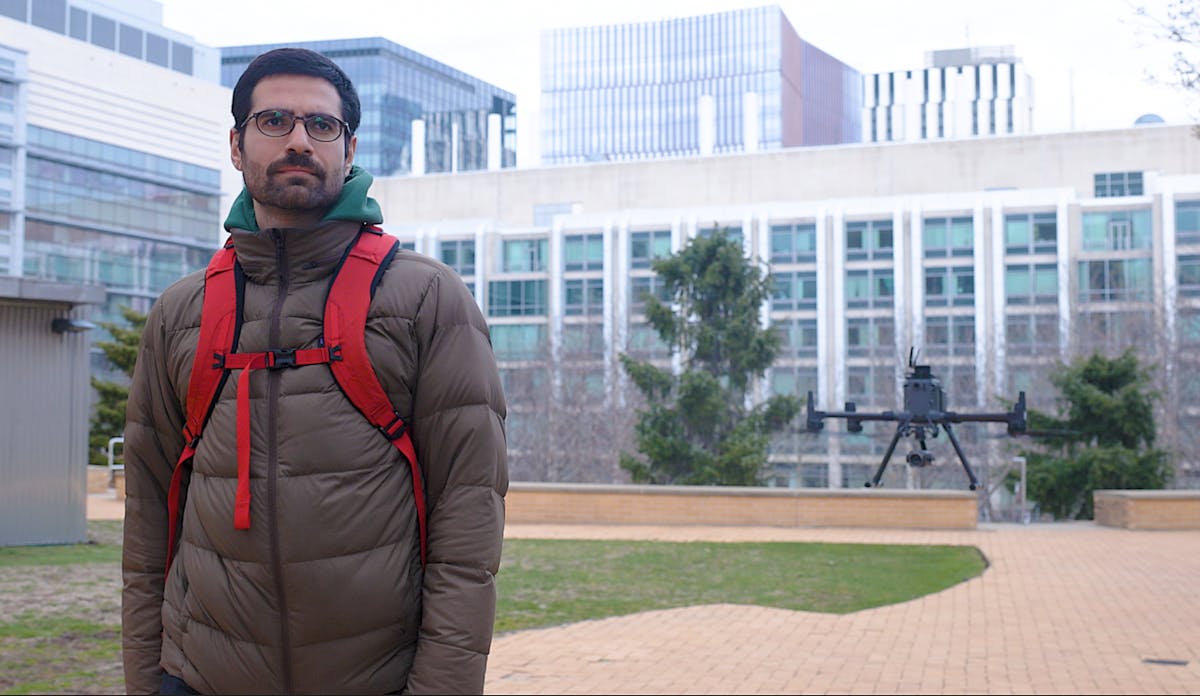
Liquid neural networks, which are a type of continuous-time recurrent neural network inspired by the brain, are constantly updated as they encounter new data. The adaptability of these models allows them to outperform other state of the art algorithms presently in use. It was discovered that in addition to improving through continuous learning, liquid neural networks also learn to distill the task they are given, focusing on important details while ignoring irrelevant information.
Similar performance could be achieved using traditional neural network architectures by scaling them up sufficiently, but only at the expense of heavy computational requirements. The amount of computer power, and energy, that can be carried on a drone is quite limited, and relying on a wireless connection to leverage cloud resources can introduce latency, or even a total loss of processing capabilities while in flight. Highly adaptable liquid networks, on the other hand, are very small by comparison, making them ideal for mobile applications.
Initial training was conducted on datasets created while a human pilot controlled a DJI M300 RTK quadcopter drone on fly-to-target tasks. The drone was equipped with a powerful NVIDIA Jetson TX2 computer to run the machine learning algorithms onboard. After collecting data from just a few runs, the system was found to be capable of adapting to drastic changes in scenery. It could successfully complete its task in environments as diverse as forests and urban landscapes, and do so across multiple seasons. These previously unseen environments were navigated with no additional offline training.
The team believes that their work could be useful in making autonomous drone deployments more efficient, cost-effective, and reliable. And that could make a wide range of applications practical, ranging from environmental monitoring and package delivery to autonomous vehicles and robotic assistants.
R&D, creativity, and building the next big thing you never knew you wanted are my specialties.