SIFTER Uses an NVIDIA Jetson Nano, Thermal Camera, and Depth Camera to Instantly Track Fevers
Able to take your temperature at up to a 10-foot range without you even noticing, SIFTER could be key to handling future pandemics.
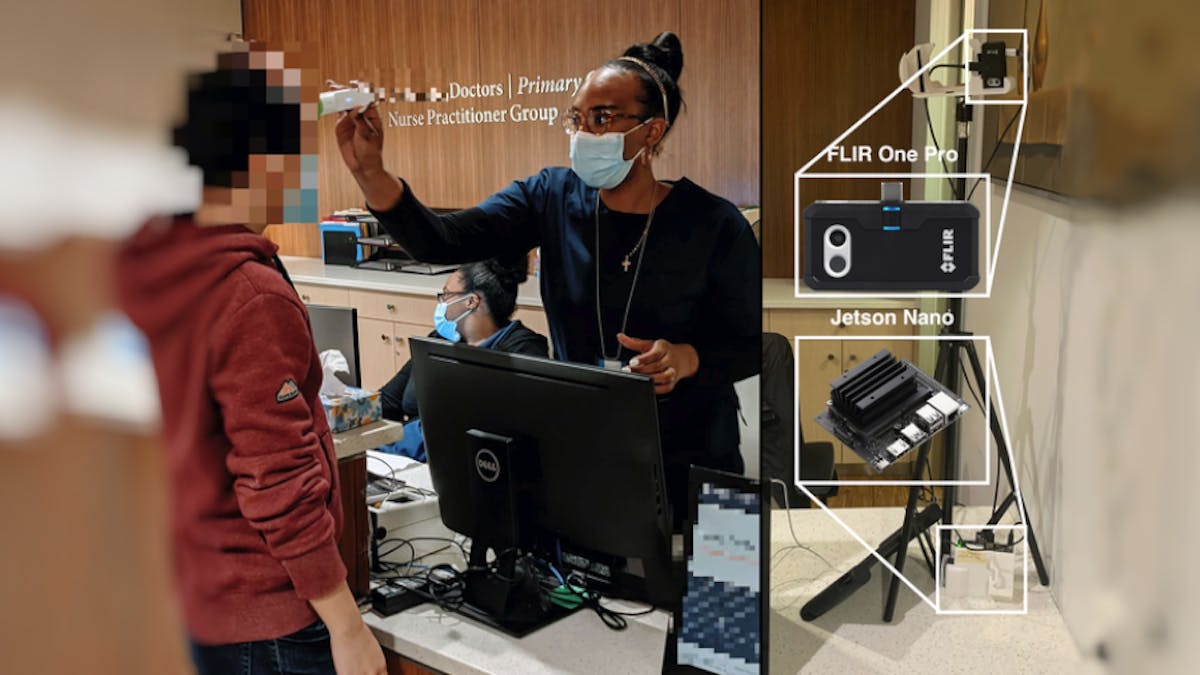
Researchers at Columbia Engineering and the Mailman School of Public Health have developed a machine learning system, which aims to make fever detection for public health as simple as possible — requiring just $500 in hardware and allowing subjects to simply walk past a checkpoint.
"By using advanced algorithms and models, we’ve developed an inexpensive way for hospitals, restaurants, subway stations, schools, hi-rise buildings with elevators, and more to perform fever screening without disrupting the normal flow of traffic, helping to restore some amount of 'normal' to our everyday lives," claims project leader Fred Jiang, associate professor of electrical engineering at Columbia.
The inspiration for the project came following Jiang's witnessing of fever scanning for visitors and patients at the hospital where his wife works. "A nurse had to scan each person in close proximity," he explains, "and it didn’t guarantee that everyone who passed through did not have fever, as people can take fever reducers."
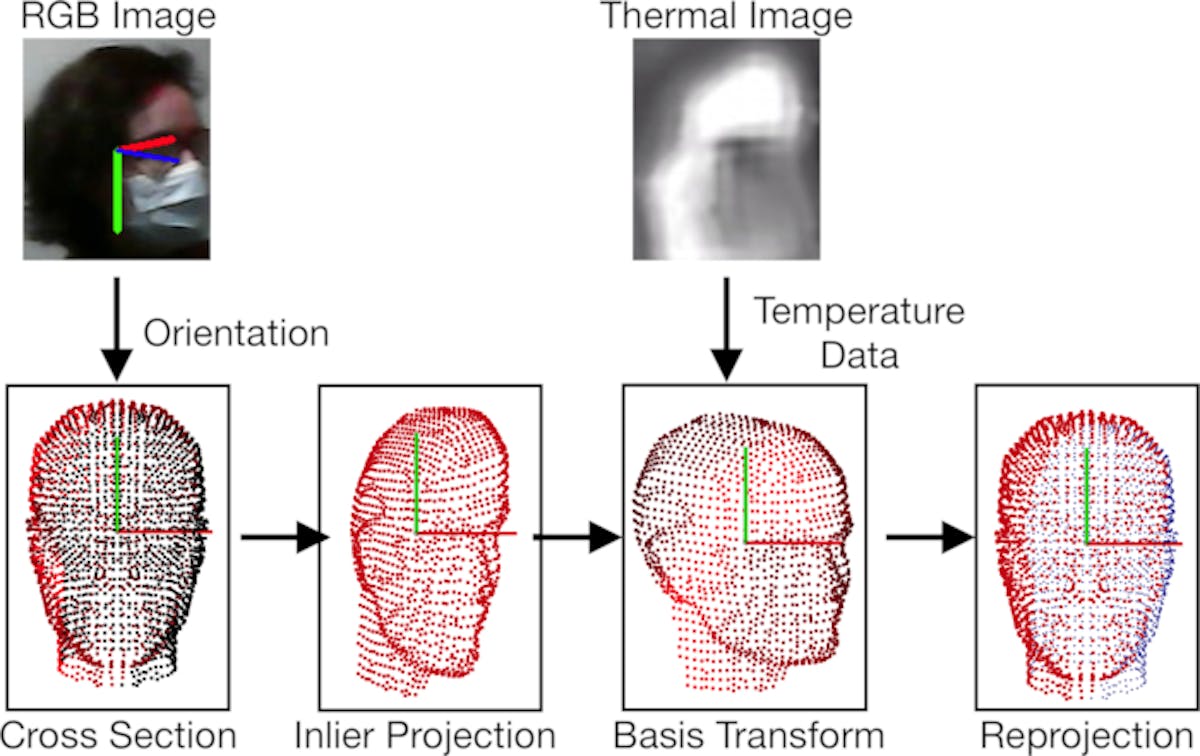
The solution: SIFTER, an open source platform that combines a sensor node with a cloud server and a web-based user interface. The sensor nodes are made up of a relatively low-cost FLIR One Pro thermal camera connected to an NVIDIA Jetson Nano alongside a depth-sensing stereo camera, which tracks heads and classifies individuals as either having a normal temperature or signs of a fever — even if they only pass by, and don't stand in front of, the sensing system, at ranges of up to three meters (around 10 feet).
The NVIDIA Jetson Nano runs a machine learning system for tracking individuals, locating their heads, estimating orientation, then capturing the thermal data as a 3D point cloud for estimation of body temperature. The distance between the sensor and the individual is measured using the depth-sensing camera, and the thermal data corrected for attenuation.
"We're very excited about SIFTER," says co-creator Andrew Rundle, professor of epidemiology. "Fever is a symptom of many infectious diseases, and this system will provide a low-cost, near-real-time and continuous means of detecting spikes in the number of people experiencing fever."
"We see SIFTER as a complement to other more costly and slower public health surveillance systems that rely on lab tests and case reporting. We expect this system to help improve detection of emerging infectious diseases and seasonal surges in flu."
More information on SIFTER is available on the project website, while the source code has been published to GitHub under the permissive MIT license. A paper on the project is to be presented this May at the 21st ACM/IEEE Conference on Information Processing in Sensor Networks (IPSN '22), but has not yet been made publicly available.
Freelance journalist, technical author, hacker, tinkerer, erstwhile sysadmin. For hire: freelance@halfacree.co.uk.