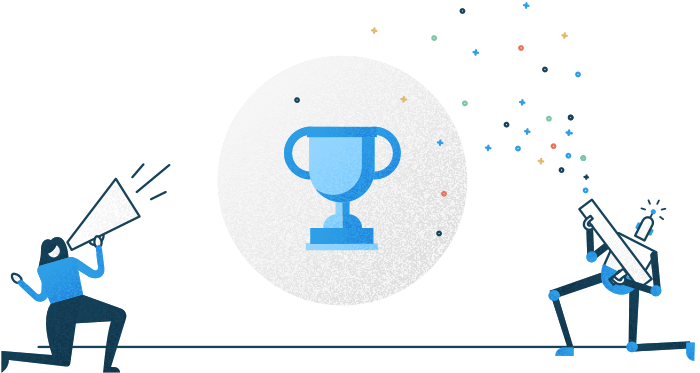
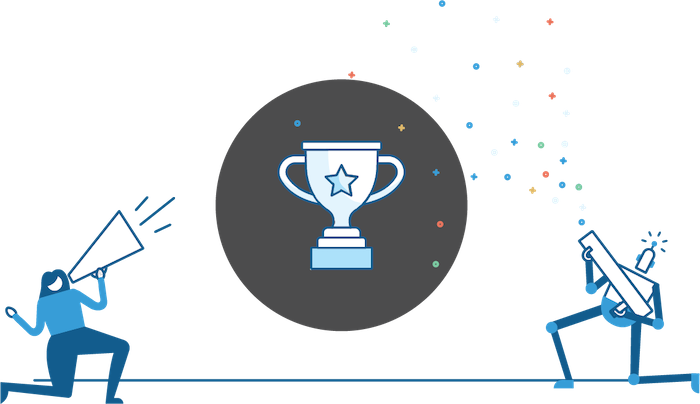
Grand Prize
Each winner was awarded a The newest Intel AI PC
Second Place Prize
Each winner was awarded an Intel ARC GPU
Honorable Mentions
Each winner was awarded a Honorable Mention
Overview
Welcome to the Visual Anomaly and Novelty Detection 2024 Challenge, VAND 2nd Edition workshop at The IEEE/CVF Conference on Computer Vision and Pattern Recognition 2024 (CVPR 2024)! This year our challenge aims to bring visual anomaly detection closer to industrial visual inspection, which has wide real-world applications. We look forward to participants from both academia and industry. Proudly sponsored by Intel, this challenge consists of two categories:
- Category 1 — Adapt & Detect: Robust Anomaly Detection in Real-World Applications
- Category 2 — VLM Anomaly Challenge: Few-Shot Learning for Logical and Structural Detection
Participants can choose a category or enter both in two separate submissions. These challenge categories aim to advance existing anomaly detection literature and increase its adaptation in real-world settings. We invite the global community of innovators, researchers, and technology enthusiasts. Engage with these challenges and contribute towards advancing anomaly detection technologies in real-world scenarios.
From April 15th - June 1st, 2024, this global community can showcase their ideas on how to solve these challenges in the visual anomaly detection field.
Category 1 — Adapt & Detect: Robust Anomaly Detection in Real-World ApplicationsIn this category, participants will develop anomaly detection models that demonstrate robustness against external factors and adaptability to real-world variability. Many existing anomaly detection models are trained on normal images and validated against normal and abnormal images. They often struggle with robustness in real-world scenarios due to data drift caused by external changes like camera angles, lighting conditions, and noise. This challenge focuses on developing models that can handle this real-world variation.
The dataset
Participants will use MVTec Anomaly Detection (MVTec AD), a public anomaly detection benchmark dataset.
Training will use the training images from these datasets. The final evaluation and leaderboard rankings will use the test sets of the respective datasets. The organizing committee will apply random perturbation to the test set to simulate the domain shift before evaluation.
Since the goal of the challenge is to make anomaly detection models robust to unknown domain shift conditions, the modified images will not be available to the participants at training time. Each included perturbation aims to simulate a type of domain shift that may occur over time in the real world. Examples are Gaussian noise to simulate camera noise or low-light conditions, brightness changes to mimic different times of day, and rotations or translations to simulate varying camera positions. The full list of perturbations will include various other perturbations which will not be made public until after the evaluation period. The perturbations will be applied in single or combinations and introduced randomly to ensure variability.
We will generate multiple versions of the test set to mitigate the risk of models achieving high scores by chance. Each version will maintain the same structure but with different perturbation seeds. This ensures that models have consistent performance across various scenarios rather than succeeding through random alignment with a particular set of perturbations. This approach challenges the models’ adaptability and robustness in environments where data characteristics may shift over time.
The model
Participants are encouraged to develop models based on the one-class training paradigm, which is training exclusively on normal images. These models are then validated and tested on a mix of normal and abnormal images to assess their anomaly detection capabilities. The focus is on enabling these models to effectively identify deviations from normality, emphasizing the real-world applicability of the techniques developed.
Evaluation
Evaluation happens on image level and pixel level F1-max scores. These use an adaptive anomaly score threshold that achieves the highest F1 metric. This approach ensures a balanced consideration of precision and recall in the models’ anomaly detection performance.
We will independently evaluate the metrics for each created test set, after which we will average scores across all categories and test sets. The final evaluation metric for each submission will be the harmonic mean of image and pixel-level F1-max scores. We average the results to guarantee a final score representing consistent performance across multiple test scenarios. This score highlights the model’s dependability in identifying abnormalities under various circumstances.
Submission Guideline
Must follow this guide.
Category 2 — VLM Anomaly Challenge: Few-Shot Learning for Logical and Structural DetectionWith the development of vision language models (VLMs), finding anomalies could reach an exciting new level, such as finding logical anomalies that need more than detecting structural defects.
Participants will create models using few-shot learning and VLMs to find and localize structural and logical anomalies. This shows that the models can handle structural defect detection and logical reasoning.
The dataset
This challenge uses the MVTec LOCO AD dataset. This dataset contains images of different industrial products, showing structural and logical anomalies.
For each few-shot learning scenario, k normal images are sampled randomly from the train set of the MVTec LOCO dataset. We will explore scenarios where k = 1, 2, 4, and 8 with the randomly selected samples provided by the organizing committee.
Additionally, if participants use text prompts within the model, they can include the name of the dataset category in their prompts.
Validation will use the validation set of the MVTec LOCO dataset.
Testing will use the dedicated test set of the MVTec LOCO dataset.
The model
Participants can pre-train their models on any public dataset except the MVTec LOCO dataset, ensuring the challenge focuses on few-shot learning capability.
Evaluation
We will follow last year’s evaluation criteria, outlined here:
- The evaluation metric for each k-shot setup in the MVTec LOCO subset will be the F1-max score for the anomaly classification task.
- We will perform three random runs using the pre-selected samples for each k-shot scenario in a particular subset. These runs will be averaged and assessed.
- The arithmetic mean of the averaged metrics is the evaluation measure for a k-normal-shot setup across each category.
- We will evaluate the effectiveness of few-shot learning algorithms by plotting the F1-max curve. This shows the F1-max scores in relation to the k-shot number. The ultimate evaluation metric will be the area under the F1-max curve (AUFC).
Submission Guideline
Must follow this guide.
Enter the Challenge: Eligibility and How to SubmitImportant Dates: Challenge time April 15th - June 1st
- Registration Opens: April 15th
- Dataset Release: April 15th
- Submission Deadline: June 1st
- Results Announcement: June 7th
Submission requirements and guidelines
- Submissions must follow a strict template and a zip file attachment in a specific format.
- Review and follow carefully the guidelines for Category 1 & Category 2 here.
- Until the submission deadline, each participant(s) can submit as many submissions as they want.
Evaluation criteria
- Reproducibility: If judges cannot reproduce the submission, the submission is disqualified.
- Evaluation metrics (100): Each category has separate evaluation metrics and will be the unique criteria judges will follow to evaluate.
- For specific details, please refer to the evaluation section of the corresponding category.
Prizes
Participate for a chance to win prizes! Prizes range from Intel Products to being featured on presentations at the CVPR workshop and opportunities for collaboration.
Grand Prize
The prestige 16” laptop is perfect for on-the-go AI-boosting performance!
- Model: MSI Prestige 16” Intel Evo Edition Laptop
- Processor: Intel Core Ultra 7
- Graphics: Intel Arc Graphics
- Memory: 32GB
- Storage: 1TB SSD
The winners will also get an invitation to present their solutions during Intel's workshop at CVPR2024!
Second Place Prize
- Model: ASRock Challenger Arc A770
- Memory: 16GB GDDR6
- Interface: PCI Express 4.0 x16
Honorable Mentions
Resources
- Join the contest discord here
- Anomalib GitHub
- OpenVINO Documenation
- Edge AI Reference Kits
Contest Status
🎉 Prizes awarded! 🎉
Timeline
Competition begins
April 15, 2024 at 1:00 PM PDT
Submissions close
June 1, 2024 at 12:00 PM PDT
Winners announced by
Jun 7, 2024